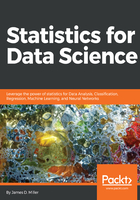
Data mining
In Chapter 1, Transitioning from Data Developer to Data Scientist, we said, with data mining, one is usually more absorbed in the data relationships (or the potential relationships between points of data, sometimes referred to as variables) and cognitive analysis.
To further define this term, we can mention that data mining is sometimes more simply referred to as knowledge discovery or even just discovery, based upon processing through or analyzing data from new or different viewpoints and summarizing it into valuable insights that can be used to increase revenue, cuts costs, or both.
Using software dedicated to data mining is just one of several analytical approaches to data mining. Although there are tools dedicated to this purpose (such as IBM Cognos BI and Planning Analytics, Tableau, SAS, and so on.), data mining is all about the analysis process finding correlations or patterns among dozens of fields in the data and that can be effectively accomplished using tools such as MS Excel or any number of open source technologies.