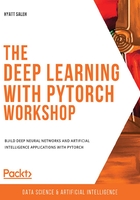
Introduction to Neural Networks
Neural networks learn from training data, rather than being programmed to solve a particular task by following a set of rules. This learning process can follow one of the following methodologies:
- Supervised learning: This is the simplest form of learning as it consists of a labeled dataset, where the neural network finds patterns that explain the relationship between the features and the target. The iterations during the learning process aim to minimize the difference between the predicted value and the ground truth. One example of this is classifying a plant based on the attributes of its leaves.
- Unsupervised learning: In contrast to the preceding methodology, unsupervised learning consists of training a model with unlabeled data (meaning that there is no target value). The purpose of this is to arrive at a better understanding of the input data. In general, networks take input data, encode it, and then reconstruct the content from the encoded version, ideally keeping the relevant information. For instance, given a paragraph, a neural network can map the words and then suggest which ones are the most important or descriptive for the paragraph. These can then be used as tags.
- Reinforcement learning: This methodology consists of learning from the input data, with the main objective of maximizing a reward function in the long run. This is achieved by learning from the data as it comes in, rather than it being trained over static data (as in supervised learning). Hence, decisions are not made based on the immediate reward, but on the accumulation of it in the entire learning process. An example of this is a model that allocates resources to different tasks, with the objective of minimizing bottlenecks that slow down general performance.
Note
From the learning methodologies we've mentioned here, the most commonly used one is supervised learning, which is the one that will be mainly used in subsequent sections. This means that all the exercises, activities, and examples in this chapter will use a labeled dataset as input data.
What Are Neural Networks?
As we discussed earlier, neural networks are a type of machine learning algorithm that's modeled on the anatomy of the human brain and that uses mathematical equations to learn a pattern from the observations that were made from the training data.
However, to actually understand the logic behind the training process that neural networks typically follow, it is important to understand the concept of perceptrons.
Developed during the 1950s by Frank Rosenblatt, a perceptron is an artificial neuron that takes several inputs and produces a binary output, similar to neurons in the human brain. This then becomes the input of a subsequent perceptron (neuron). Perceptrons are the essential building blocks of a neural network (just like neurons are the building blocks of the human brain):

Figure 2.1: Diagram of a perceptron
Here, X1, X2, X3, and X4 represent the different inputs of the perceptron, and there could be any number of these. The circle is the perceptron, which is where the inputs are processed to arrive at an output.
Rosenblatt also introduced the concept of weights (w1, w2, …, wn), which are numbers that express the importance of each input. The output can be either 0 or 1, and it depends on whether the weighted sum of the inputs is above or below a given threshold (a numerical limit set by the developer or by a constraint of the data problem), which can be set as a parameter of the perceptron, as can be seen here:

Figure 2.2: Equation for the output of perceptrons
Exercise 2.01: Performing the Calculations of a Perceptron
The following exercise does not require programming of any kind; instead, it consists of simple calculations to help you understand the notion of the perceptron. To perform these calculations, consider the following scenario.
There is a music festival in your town next Friday, but you are ill and trying to decide whether to go (where 0 means you are not going and 1 means you are going). Your decision relies on three factors:
- Will there be good weather? (X1)
- Do you have someone to go with? (X2)
- Is the music to your liking? (X3)
For the preceding factors, we will use 1 if the answer to the question is yes, and 0 if the answer is no. Additionally, since you are very sick, the factor related to the weather is highly relevant, and you decide to give this factor a weight twice as big as the other two factors. Hence, you decide that the weights for the factors will be 4 (w1), 2 (w2), and 2 (w3). Now, consider a threshold of 5:
- With the information provided, calculate the output of the perceptron when considering that the weather is not good next Friday, but that you have someone to go with and you like the music at the festival:

Figure 2.3: Output of the perceptron
Considering that the output is less than the threshold, the final result will be equal to 0, meaning that you should not go to the festival to avoid the risk of getting even more ill.
You have successfully performed the calculations of a perceptron, which is the starting point of understanding the learning process that occurs inside neural networks.
Multi-Layer Perceptron
Considering what we learned about in the previous section, the notion of a multi-layered network consists of a network of multiple perceptrons stacked together (also known as nodes or neurons), such as the one shown here:

Figure 2.4: Diagram of a multi-layer perceptron
Note
The conventional way to refer to the layers in a neural network is as follows:
The first layer is the input layer, the last layer is the output layer, and all the layers in between are hidden layers.
Here, again, a set of inputs is used to train the model, but instead of feeding a single perceptron, they are fed to all the perceptrons (neurons) in the first layer. Next, the outputs that are obtained from this layer are used as inputs for the perceptrons in the subsequent layer and so on until the final layer is reached, which is in charge of outputting a result.
Note that the first layer of a perceptron handles a simple decision process by weighting the inputs, while the subsequent layer can handle more complex and abstract decisions based on the output of the previous layer, and hence the state-of-the-art performance of deep neural networks (networks that use many layers) for complex data problems.
Different to conventional perceptrons, neural networks have evolved to have one or multiple nodes in the output layer so that they are able to present the result either as binary or multiclass.
The Learning Process of a Neural Network
In general terms, a neural network is made up of multiple neurons, where each neuron computes a linear function, along with an activation function, to arrive at an output based on some inputs (an activation function is designed to break linearity – this will be explained in more detail later in this chapter). This output is tied to a weight, which represents its level of importance, and will be used for calculations in the following layer.
Moreover, these calculations are carried out throughout the entire architecture of the network, until a final output is reached. This output is used to determine the performance of the network in comparison to the ground truth, which is then used to adjust the different parameters of the network to start the calculation process over again.
Considering this, the training process of a neural network can be seen as an iterative process that goes forward and backward through the layers of the network to arrive at an optimal result, which can be seen in the following diagram (loss functions will be covered later in this chapter):

Figure 2.5: Diagram of the learning process of a neural network
Forward Propagation
This is the process of going from left to right through the architecture of the network while performing calculations using the input data to arrive at a prediction that can be compared to the ground truth. This means that every neuron in the network will transform the input data (the initial data or data received from the previous layer) according to the weights and biases that it has associated with it and will send the output to the subsequent layer until a final layer is reached and a prediction is made.
Note
In neural networks, biases are numerical values that help shift the activation function of each neuron in order to avoid zero values that may affect the training process. Their role in the training of neural networks will be explained later in this chapter.
The calculations that are performed in each neuron include a linear function that multiplies the input data by some weight plus a bias, which is then passed through an activation function. The main purpose of the activation function is to break the linearity of the model, which is crucial considering that most real-life data problems that are solved using neural networks are not defined by a line, but rather by a complex function. These formulas are as follows:

Figure 2.6: Calculations performed by each neuron
Here, as we mentioned previously, X refers to the input data, W is the weight that determines the level of importance of the input data, b is the bias value, and sigma () represents the activation function that's applied over the linear function.
The activation function serves the purpose of introducing non-linearity to the model. There are different activation functions to choose from, and a list of the ones most commonly used nowadays is as follows:
- Sigmoid: This is S-shaped, and it basically converts values into simple probabilities between 0 and 1, where most of the outputs that are obtained by the sigmoid function will be close to the extremes of 0 and 1:

Figure 2.7: Sigmoid activation function
The following plot shows the graphical representation of the sigmoid activation function:

Figure 2.8: Graphical representation of the sigmoid activation function
- Softmax: Similar to the sigmoid function, this calculates the probability distribution of an event over n events, meaning that its output is not binary. In simple terms, this function calculates the probability of the output being one of the target classes in comparison to the other classes:

Figure 2.9: Softmax activation function
Considering that its output is a probability, this activation function is often found in the output layer of classification networks.
- Tanh: This function represents the relationship between the hyperbolic sine and the hyperbolic cosine, and the result is between -1 and 1. The main advantage of this activation function is that negative values can be dealt with more easily:

Figure 2.10: Tanh activation function
The following plot shows the graphical representation of the tanh activation function:

Figure 2.11: Graphical representation of the tanh activation function
- Rectified Linear Function (ReLU): This basically activates a node given that the output of the linear function is above 0; otherwise, its output will be 0. If the output of the linear function is above 0, the result from this activation function will be the raw number it received as input:

Figure 2.12: ReLU activation function
Conventionally, this activation function is used for all hidden layers. We will learn more about hidden layers in the upcoming sections of this chapter. The following plot shows the graphical representation of the ReLU activation function:

Figure 2.13: Graphical representation of the ReLU activation function
The Calculation of Loss Functions
Once forward propagation is complete, the next step in the training process is to calculate a loss function to estimate the error of the model by comparing how good or bad the prediction is in relation to the ground truth value. Considering this, the ideal value to be reached is 0, which would mean that there is no pergence between the two values.
This means that the goal in each iteration of the training process is to minimize the loss function by changing the parameters (weights and biases) that are used to perform the calculations during the forward pass.
Again, there are multiple loss functions to choose from. However, the most commonly used loss functions for regression and classification tasks are as follows:
- Mean squared error (MSE): Widely used to measure the performance of regression models, the MSE function calculates the sum of the distance between the ground truth and the prediction values:

Figure 2.14: MSE loss function
Here, n refers to the number of samples, is the ground truth values, and
is the predicted value.
- Cross-entropy/multi-class cross-entropy: This function is conventionally used for binary or multi-class classification models. It measures the pergence between two probability distributions; a large loss function will represent a large pergence. Hence, the objective here is to also minimize the loss function:

Figure 2.15: Cross-entropy loss function
Again, n refers to the number of samples. and
are the ground truth and the predicted value, respectively.
Backward Propagation
The final step in the training process consists of going from right to left in the architecture of the network to calculate the partial derivatives (also known as gradients) of the loss function in respect to the weights and biases in each layer in order to update these parameters (weights and biases) so that in the next iteration step, the loss function is lower.
The final objective of the optimization algorithm is to find the global minima where the loss function has reached the least possible value, as shown in the following plot:
Note
A local minima refers to the smallest value within a section of the function domain. On the other hand, a global minima refers to the smallest value of the entire domain of the function.

Figure 2.16: Loss function optimization through the iteration steps in a two-dimensional space
Here, the dot furthest to the left, A, is the initial value of the loss function before any optimization. The dot furthest to the right, B, at the bottom of the curve, is the loss function after several iteration steps, where its value has been minimized. The process of going from one dot to another is called a step.
However, it is important to mention that the loss function is not always as smooth as the preceding one, which can introduce the risk of reaching a local minima during the optimization process.
This process is also called optimization, and there are different algorithms that vary in methodology to achieve the same objective. The most commonly used optimization algorithm will be explained next.
Gradient Descent
Gradient descent is the most widely used optimization algorithm among data scientists, and it is the basis of many other optimization algorithms. After the gradients for each neuron are calculated, the weights and biases are updated in the opposite direction of the gradient, which should be multiplied by a learning rate (used to control the size of the steps taken in each optimization), as shown in the following equations.
The learning rate is crucial during the training process as it prevents the update of the weights and biases from over/undershooting, which may prevent the model from reaching convergence or delay the training process, respectively.
The optimization of weights and biases in the gradient descent algorithm is as follows:

Figure 2.17: Optimization of parameters in the gradient descent algorithm
Here, α refers to the learning rate, and dw/db represents the gradients of the weights or biases in a given neuron. The product of the two values is subtracted from the original value of the weight or bias in order to penalize the higher values, which are contributing to computing a large loss function.
An improved version of the gradient descent algorithm is called stochastic gradient descent, and it basically follows the same process, with the distinction that it takes the input data in random batches instead of in one chunk, which improves the training times while reaching outstanding performance. Moreover, this approach allows for the use of larger datasets because by using small batches of the dataset as inputs, we are no longer limited by computational resources.
Advantages and Disadvantages
The following is an explanation of the advantages and disadvantages of neural networks.
Advantages
Neural networks have become increasingly popular in the last few years for four main reasons:
- Data: Neural networks are widely known for their ability to capitalize on large amounts of data, and thanks to the advances in hardware and software, the collection and storage of massive databases is now possible. This has allowed neural networks to show their real potential as more data is fed into them.
- Complex data problems: As we explained previously, neural networks are excellent for solving complex data problems that cannot be tackled by other machine learning algorithms. This is mainly due to their ability to process large datasets and uncover complex patterns.
- Computational power: Advances in technology have also increased the computational power that's available these days, which is crucial for training neural network models that use millions of pieces of data.
- Academic research: Thanks to the preceding three points, a proliferation of academic research on this topic is available on the internet, which not only facilitates the immersion of new research each day, but also helps keep the algorithms and hardware/software requirements up to date.
Disadvantages
Just because there are a lot of advantages to using a neural network does not mean that every data problem should be solved this way. This is a mistake that is commonly made. There is no one algorithm that will perform well for all data problems, and selecting the algorithm to use should depend on the resources available, as well as the data problem.
Although neural networks are thought to outperform almost any machine learning algorithm, it is crucial to consider their disadvantages as well so that you can weigh up what matters most for the data problem. Let's go through them now:
- Black box: This is one of the most commonly known disadvantages of neural networks. It basically means that how and why a neural network reached a certain output is unknown. For instance, when a neural network incorrectly predicts a cat picture as a dog, it is not possible to know what the cause of the error was.
- Data requirements: The vast amounts of data that they require to achieve optimal results can be equally an advantage and a disadvantage. Neural networks require more data than traditional machine learning algorithms, which can be the main reason to choose between them and other algorithms for some data problems. This becomes a greater issue when the task at hand is supervised, which means that the data needs to be labeled.
- Training times: Tied to the preceding disadvantage, the need for vast amounts of data also makes the training process last longer than traditional machine learning algorithms, which, in some cases, is not an option. Training times can be reduced through the use of GPUs, which speed up computation.
- Computationally expensive: Again, the training process of neural networks is computationally expensive. While one neural network could take weeks to converge, other machine learning algorithms could take hours or minutes to be trained. The amount of computational resources needed depends on the quantity of data at hand, as well as the complexity of the network; deeper neural networks take a longer time to train.
Note
There are a wide variety of neural network architectures. Three of the most commonly used ones will be explained in this chapter, along with their practical implementation in subsequent chapters. However, if you wish to learn about other architectures, visit http://www.asimovinstitute.org/neural-network-zoo/.
Introduction to Artificial Neural Networks
Artificial neural networks (ANNs), also known as multi-layer perceptrons, are collections of multiple perceptrons. The connection between perceptrons occurs through layers. One layer can have as many perceptrons as desired, and they are all connected to all the other perceptrons in the preceding and subsequent layers.
Networks can have one or more layers. Networks with over four layers are considered to be deep neural networks and are commonly used to solve complex and abstract data problems.
ANNs are typically composed of three main elements, which were explained earlier, and can also be seen in the following image:
- Input layer: This is the first layer of the network, conventionally located furthest to the left in the graphical representation of a network. It receives the input data before any calculation is performed and completes the first set of calculations. This is where the most generic patterns are uncovered.
For supervised learning problems, the input data consists of a pair of features and targets. The job of the network is to uncover the correlation or dependency between the features and target.
- Hidden layers: Next, the hidden layers can be found. A neural network can have many hidden layers, meaning there can be any number of layers between the input layer and the output layer. The more layers it has, the more complex data problems it can tackle, but it will also take longer to train. There are also neural network architectures that do not contain hidden layers at all, which is the case with single-layer networks.
In each layer, a computation is performed based on the information that's received as input from the previous layer, which is then used to output a value that will become the input of the subsequent layer.
- Output layer: This is the last layer of the network as is located at the far right of the graphical representation of the network. It receives data after the data has been processed by all the neurons in the network to make a final prediction.
The output layer can have one or more neurons. The former refers to models where the solution is binary, in the form of 0s or 1s. On the other hand, the latter case consists of models that output the probability of an instance belonging to each of the possible class labels (the possible values that the target variable has), meaning that the layer will have as many neurons as there are class labels:

Figure 2.18: Architecture of a neural network with two hidden layers
Introduction to Convolutional Neural Networks
Convolutional Neural Networks (CNNs) are mostly used in the field of computer vision, where, in recent decades, machines have achieved levels of accuracy that surpass human ability.
CNNs create models that use subgroups of neurons to recognize different aspects of an image. These groups should be able to communicate with each other so that, together, they can form the complete image.
Considering this, the layers in the architecture of a CNN pide their recognition tasks. The first layers focus on trivial patterns, while the layers at the end of the network use that information to uncover more complex patterns.
For instance, when recognizing human faces in pictures, the first couple of layers focus on finding edges that separate one feature from another. Next, the subsequent layers emphasize certain features of the face, such as the nose. Finally, the last couple of layers use this information to put the entire face of the person together.
This idea of activating a group of neurons when certain features are encountered is achieved through the use of filters (kernels), which are one of the main building blocks of the architecture of CNNs. However, they are not the only elements present in the architecture, which is why a brief explanation of all the components of CNNs will be provided here:
Note
The concepts of padding and stride, which you might have heard of when using CNNs, will be explained in subsequent chapters of this book.
- Convolutional layers: In these layers, a convolutional computation occurs between an image (represented as a matrix of pixels) and a filter. This computation produces a feature map as output that ultimately serves as input for the next layer.
The computation takes a subsection of the image matrix of the same shape of the filter and performs a multiplication of the values. Then, the sum of the product is set as the output for that section of the image, as shown in the following diagram:
Figure 2.19: Convolution operation between the image and filter
Here, the matrix to the left is the input data, the matrix in the middle is the filter, and the matrix to the right is the output from the computation. The computation that occurred with the values highlighted by the boxes can be seen here:
Figure 2.20: Convolution of the first section of the image
This convolutional multiplication is done for all the subsections of the image. The following diagram shows another convolution step for the same example:
Figure 2.21: A further step in the convolution operation
One important notion of convolutional layers is that they are invariant in such a way that each filter will have a specific function, which does not vary during the training process. For instance, a filter in charge of detecting ears will only specialize in that function throughout the training process.
Moreover, a CNN will typically have several convolutional layers, considering that each of them will focus on identifying a particular feature or set of features of the image, depending on the filters that are used. Commonly, there is one pooling layer between two convolutional layers.
- Pooling layers: Although convolutional layers are capable of extracting relevant features from images, their results can become enormous when analyzing complex geometrical shapes, which would make the training process impossible in terms of computational power, hence the invention of pooling layers.
These layers not only accomplish the goal of reducing the output of the convolutional layers, but also achieve the removal of any noise that's present in the features that have been extracted, which ultimately helps to increase the accuracy of the model.
There are two main types of pooling layers that can be applied, and the idea behind them is to detect the areas that express a stronger influence in the image so that the other areas can be overlooked.
Max pooling: This operation consists of taking a subsection of the matrix of a given size and taking the maximum number in that subsection as the output of the max pooling operation:
Figure 2.22: A max pooling operation
In the preceding diagram, by using a 3 x 3 max pooling filter, the result on the right is achieved. Here, the yellow section (top-left corner) has a maximum number of 4, while the orange section (top-right corner) has a maximum number of 5.
Average pooling: Similarly, the average pooling operation takes subsections of the matrix and takes the number that meets the rule as output, which, in this case, is the average of all the numbers in the subsection in question:
Figure 2.23: An average pooling operation
Here, using a 3 x 3 filter, we get 2.9, which is the average of all the numbers in the yellow section (top-left corner), while 3.2 is the average for the ones in the orange section (top-right corner).
- Fully connected layers: Finally, considering that the network would be of no use if it was only capable of detecting a set of features without having the capability of classifying them into a class label, fully connected layers are used at the end of CNNs to take the features that were detected by the previous layer (known as the feature map) and output the probability of that group of features belonging to a class label, which is used to make the final prediction.
Like ANNs, fully connected layers use perceptrons to calculate an output based on a given input. Moreover, it is crucial to mention that CNNs typically have more than one fully connected layer at the end of the architecture.
By combining all of these concepts, the conventional architecture of CNNs is obtained. There can be as many layers of each type as desired, and each convolutional layer can have as many filters as desired (each for a particular task). Additionally, the pooling layer should have the same number of filters as the previous convolutional layer, as shown in the following image:

Figure 2.24: Diagram of the CNN architecture
Introduction to Recurrent Neural Networks
The main limitation of the aforementioned neural networks (ANNs and CNNs) is that they learn only by considering the current event (the input that is being processed) without taking into account previous or subsequent events, which is inconvenient considering that we humans do not think that way. For instance, when reading a book, you can understand each sentence better by considering the context from the previous paragraph or more.
Due to this, and taking into account the fact that neural networks aim to optimize several processes that are traditionally done by humans, it is crucial to think of a network that's able to consider a sequence of inputs and outputs, hence the creation of recurrent neural networks (RNNs). They are a robust type of neural network that allow solutions to be found for complex data problems through the use of internal memory.
Simply put, these networks contain loops in them that allow for the information to remain in their memory for longer periods, even when a subsequent set of information is being processed. This means that a perceptron in an RNN not only passes over the output to the following perceptron, but it also retains a bit of information to itself, which can be useful for analyzing the next bit of information. This memory-keeping capability allows them to be very accurate in predicting what's coming next.
The learning process of an RNN, similar to other networks, tries to map the relationship between an input (x) and an output (y), with the difference being that these models also take into consideration the entire or partial history of previous inputs.
RNNs allow sequences of data to be processed in the form of a sequence of inputs, a sequence of outputs, or even both at the same time, as shown in the following diagram:

Figure 2.25: Sequence of data handled by RNNs
Here, each box is a matrix and the arrows represent a function that occurs. The bottom boxes are the inputs, the top boxes are the outputs, and the middle boxes represent the state of the RNN at that point, which holds the memory of the network.
From left to right, the preceding diagrams can be explained as follows:
- A typical model that does not require an RNN to be solved. It has a fixed input and a fixed output. This can refer to image classification, for instance.
- This model takes in an input and yields a sequence of outputs. Take, for instance, a model that receives an image as input; the output should be an image caption.
- Contrary to the preceding model, this model takes a sequence of inputs and yields a single outcome. This type of architecture can be seen on sentiment analysis problems, where the input is the sentence to be analyzed and the output is the predicted sentiment behind the sentence.
- The final two models take a sequence of inputs and return a sequence of outputs, with the difference being that the first one analyzes the inputs and generates the outputs at the same time; for example, when each frame of a video is being labeled inpidually. On the other hand, the second many-to-many model analyzes the entire set of inputs in order to generate the set of outputs. An example of this is language translation, where the entire sentence in one language needs to be understood before proceeding with the actual translation.